Digital twin developed for resistive random access memory (RRAM)
Advancements in neuromorphic computing (NC) hardware are essential for the development of efficient and scalable artificial intelligence systems, particularly in optimizing components that can emulate biological synaptic behavior. This research is significant as it addresses the optimization of memristive components and programming schemes for use as artificial synapses within (spiking) neural networks—an approach crucial for enhancing processing efficiency and energy management in these systems.
In a collaborative effort by IHP Frankfurt (Oder), BTU Cottbus-Senftenberg, Forschungszentrum Jülich, and additional partners, a digital twin for resistive random-access memory (RRAM) devices was developed and recently published in Scientific Reports ( https://doi.org/10.1038/s41598-024-73439-z). The digital twin model is based on empirical data, capturing and replicating variations in resistance states. Experimental data collected by PGI-7/NEUROTEC scientists are loaded to automatically create the digital twin, which is seamlessly integrated into a system-level simulator. This innovation enables the accurate performance evaluation of RRAM devices under inference workloads, paving the way for more effective and energy-efficient neural network hardware solutions.
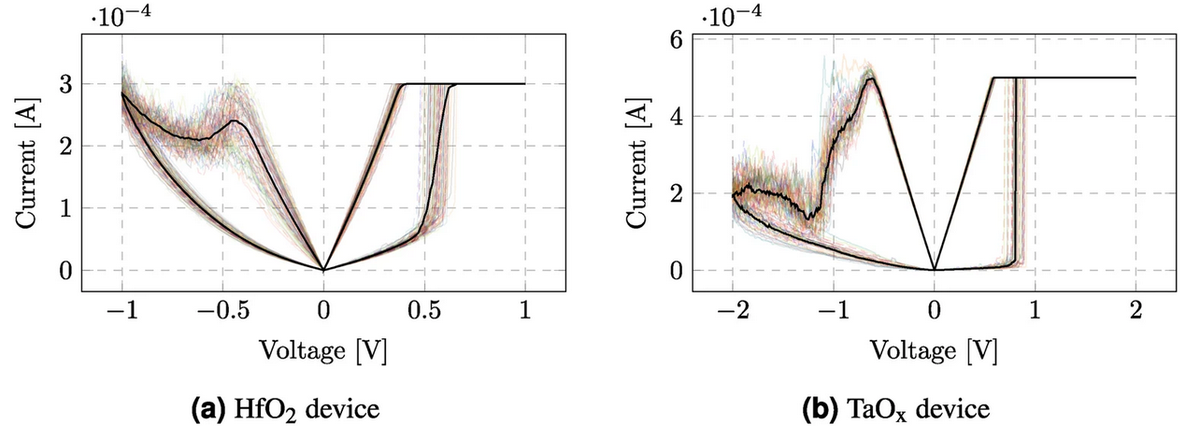